- OptiMAIL
- Posts
- 🧑💻📈 - What Values Are Embedded in AI Technology?
🧑💻📈 - What Values Are Embedded in AI Technology?
AI Ethics: 3 Core Fundamentals to Understand.
Introduction
“Those who take the time to understand how Artificial Intelligence works will end up inheriting the Earth.” - Nigel Toon.
“This background knowledge will also help us understand why artificial intelligence is not going to run out of control.” - Nigel Toon again!
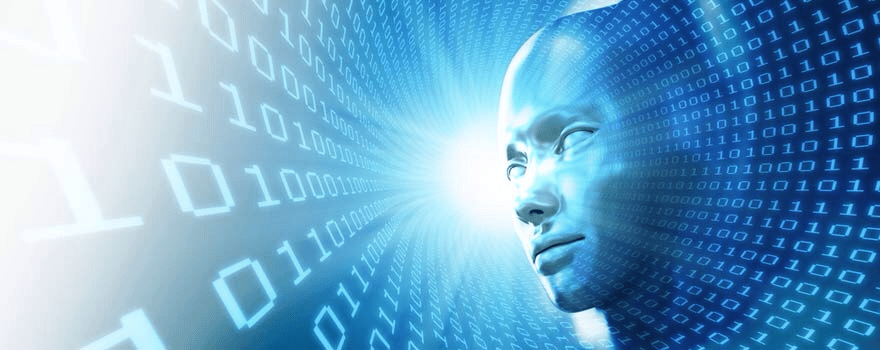
Understanding ethical considerations is a cornerstone of understanding AI.
I will explain 3 core fundamentals of AI ethics in design; their meaning, the principles in practice and why they matter to you.
Transparency.
Fairness.
Accountability.
As a reader of this newsletter, you’re very smart. You will understand the meaning of all those words in a vacuum.
By the end of this newsletter you will also understand them in the AI context.
Transparency:

Meaning:
AI systems can sometimes function as "black boxes."
This implies that their decision-making processes could be opaque to users and/or developers.
Transparency brings openness about the system's operations.
This in turn makes what it’s doing clear to users, developers, and stakeholders.
Explaining these processes is vital for building trust with the end-users.
Principles in Practice:
Making AI models open for scrutiny can help identify potential flaws and biases - more on this shortly. This enhances both transparency and reliability.
A great example of this is Google DeepMind.
They have committed to sharing a great amount of their work with the academic community.
Why transparency matters to you?
Clear communication enables users to comprehend how AI affects them.
This comprehension leads to a more informed public and an enhanced user experience.
Transparency fosters trust in the system.
Whether you are developing your own AI systems for users, or utilising commercial tools yourself.
Fairness:

Meaning:
Fairness refers to the ability of AI systems to be fair - obviously - and free from societal bias.
This is crucial.
AI applications can sometimes reflect or amplify bias present in the training data or design.
Review any data you're using for its fairness.
This helps in preventing any form of discrimination that might arise from biased algorithms.
Principles in Practice:
There are techniques you can implement to promote fairness.
Use of balanced data sets.
Applying fairness algorithms.
Monitoring to ensure unbiased results.
IBM's Fairness 360 toolkit is an example of this.
It provides developers with the necessary tools to detect and mitigate bias in their AI systems.
This open-source toolkit encourages broader adoption of fairness principles in AI development.
Why Fairness Matters to You:
Fair AI systems grow an inclusive digital environment, aiming to reflect societal values of equality and justice.
By ensuring fairness, companies abide by ethical standards and build trust in AI technologies.
Fairness in AI is also a business priority.
Biased systems - yours or otherwise - can lead to a loss of customer trust. It could cause potential legal challenges, and harm to the brand's reputation.
Fair AI promotes a positive user experience and broadens market reach by appealing to diverse user groups.